In a new report distributed in Nature Medication, scientists fostered a profound learning approach for cancer beginning separation utilizing cytological histology (Light), perceiving harm and anticipating growth beginning in hydrothorax and ascites utilizing cytological pictures from 57,220 patients.
Foundation
Histopathology diagnoses CUP tumors as metastases, but conventional methods can’t determine their origin.
These diseases often present as serous emanations and have a bleak guess regardless of mixed chemotherapies. Immunohistochemistry predicts the most probable beginning of CUP; notwithstanding, scientists can recognize a couple of cases utilizing immunostaining mixed drinks. The exact recognizable proof of essential destinations is basic for effective and customized treatment.
About the review
In the current review, specialists present Light, a profound learning calculation, to distinguish disease beginning given cytological pictures from ascites and hydrothorax.
The analysts prepared the model utilizing four free profound brain networks consolidated to deliver 12 distinct models. Utilizing cytological pictures, the scientists endeavored to foster a computerized reasoning-based demonstrative model for foreseeing cancer beginning among people with threat and ascites or hydrothorax metastases.
They tried and affirmed the computer-based intelligence framework’s presentation utilizing cytological smear occurrences from various free testing sets.
Between June 2010 and October 2023, analysts collected data from 90,572 cytological smear images of 76,183 cancer patients across four institutions.
Respiratory problems addressed the most noteworthy rate (30%, 17,058 patients) of dangerous groupings. Carcinoma represented 57% of ascites and hydrothorax cases, with adenocarcinoma being the most widely recognized bunch (47%, 27,006 patients). Just 0.6% of the squamous cell carcinomas metastasized to ascites or pleural emanation (n=346).
To validate Light’s reliability, 4,520 patients from Tianjin Cancer Clinic and 12,467 from Yantai Clinic were included.
They arbitrarily chose 496 cytology smear pictures from three inside testing sets to explore whether Light could assist junior pathologists with working on their presentation.
They analyzed the lesser pathologists’ exhibition involving Light to earlier manual translation results for both junior and more seasoned pathologists.
Scientists utilized consideration heatmaps to decipher an artificial intelligence model for disease recognition in 42,682 cytological smear pictures from patients at three significant tertiary reference emergency clinics. The model was assessed in genuine situations using outer testing datasets, which included 495 photographs.
The review plans to upgrade junior pathologists’ demonstrative capacities utilizing Light. Removal tests evaluated the benefits of remembering clinical qualities for cancer beginning expectations and examined the relationship between clinical variables and cytological pictures.
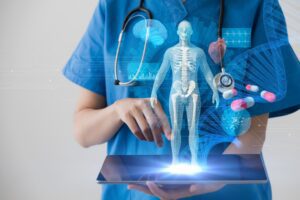
Results
Researchers evaluated the Light model, a clever procedure for predicting cancer origins in diagnosis and treatment, across various datasets.
Light’s findings: AUROC 0.97, 83% accuracy for top 1, surpassing pathologists.
When combined with five testing sets, Light had a best 1 exactness of 83%, a main 2 precision of 96%, and a best 3 precision of close to 100%. It likewise created a comparative miniature arrived at the midpoint of one-versus-rest AUROC evaluations in the low-sureness and high-assurance gatherings.
The review included 391 disease patients, of which 276 were concordant and 115 harsh. After the subsequent period, 42% of the patients passed on, with 37% concordant patients and 53% dissonant ones. Endurance examination uncovered that concordant patients had extensively higher general endurance than harsh ones.
Image quality issues like segment folding, impurities, over-staining can contribute to AI overdiagnosis in pancreatic cancer. Scientists can address these blemishes by fastidious manual handling all through the information screening step.
In cases of colonic cancer, sludge occupied a significant portion of the image, potentially leading the AI model to miss crucial details.
End
In light of the review discoveries, the Light model, a simulated intelligence device, has shown a guarantee in clinical practice for anticipating the essential framework beginning of threatening cells in hydrothorax and ascites.
It can recognize threatening growths and harmless diseases, pinpoint malignant growth sources, and help in clinical dynamics in patients with tumors of obscure beginning. The model performed well across five testing sets and outflanked four pathologists.
It can help oncologists in choosing treatment for unidentified people with CUP, basically adenocarcinoma, treated with experimental expansive-range chemotherapy regimens.